[cjtoolbox name=”Paper Kit – JS”][/cjtoolbox]
Room | |||||
Monday, Oct. 27 | Dupin 1 | Dupin 2 | Dickens 1+2 | Dickens 3+4 | Dickens 5+6 |
Morning | T1 | T2 | T3 | T4 | T5 |
Afternoon | T9 | T10 | T6 | T7 | T8 |
Speakers: Jean-Christophe Olivo-Marin (Institut Pasteur), Habib Zaidi (Geneva University Hospital, Groningen University)
Location: Dupin 1
Description: This tutorial comprises two parts presented by two speakers as follows:
Part 1—One major open problem in biological research is the quantification of dynamics parameters and the characterization of phenotypic and morphological changes occurring as a consequence of such diverse events such as cell motility, host/pathogen interaction, organism development or social interactions between animals. An increasing number of biological projects aim indeed at elucidating the links between biological function and phenotype through imaging and modelling the spatiotemporal characteristics of cellular or organism dynamics. This part of the tutorial will present and discuss some recent developments of algorithms and software for robust quantitative assessment of 2D/3D+t dynamic bioimaging data. It will also demonstrate on a number of examples how the use of these tools to extract automatically quantitative data from bioimages has enabled the understanding of biological information contained therein. Participants in the tutorial will learn how bioimaging and bioimage analysis are used to uncover biological mechanisms and functions, and why algorithms and computational techniques have enabled these discoveries. They will also learn more about open-source software platforms in bioimaging and their impact on research.
Part 2—This part of the tutorial reflects the tremendous increase in interest in molecular and dual-modality imaging (PET/CT, SPECT/CT and PET/MR) as both clinical and research imaging modalities in the past decade. An overview of molecular mutli-modality medical imaging instrumentation as well as simulation, reconstruction, quantification and related image-processing issues with special emphasis on quantitative analysis of nuclear medical images are presented. This tutorial aims to bring to the biomedical image-processing community a review of state-of-the-art algorithms not only in current use but also under development for accurate quantitative analysis in multimodality and multiparametric molecular imaging. The tutorial will also cover algorithm validation mainly from the developer’s perspective with emphasis on image-reconstruction and analysis techniques. It will inform the audience about a series of advanced development recently carried out at the PET Instrumentation & Neuroimaging Lab of Geneva University Hospital and other active research groups. Current and prospective future applications of quantitative molecular imaging are also addressed, especially its use prior to therapy for dose distribution modelling and optimisation of treatment volumes in external radiation therapy and patient-specific 3D dosimetry in targeted therapy towards the concept of image-guided radiation therapy.
Biographies:
Jean-Christophe Olivo-Marin received the PhD and the HDR degrees in optics and signal processing from the Institut d’Optique Théorique et Appliquée, University of Paris-Orsay, France. He is the head of the Quantitative Image Analysis Unit, Institut Pasteur, and the chair of the Cell Biology and Infection Department. He was a cofounder of the Institut Pasteur Korea, Seoul, where he held a joint appointment as a chief technology officer from 2004 to 2005. Previous to that, he was a staff scientist at the European Molecular Biology Laboratory, Heidelberg, from 1990 to 1998. His research interests are in image analysis of multidimensional microscopy images, computer vision and motion analysis for cellular dynamics, and in multidisciplinary approaches for biological imaging. He is a fellow of the IEEE, past chair of the IEEE SPS Bio Imaging and Signal Processing Technical Committee (BISP-TC), a senior area editor of the IEEE Signal Processing Letters, and a member of the Editorial Board of the journals Medical Image Analysis and BMC Bioinformatics. He was the general chair of the IEEE International Symposium on Biomedical Imaging in 2008.
Habib Zaidi is Chief Physicist and head of the PET Instrumentation & Neuroimaging Laboratory at Geneva University Hospital and faculty member at the medical school of Geneva University. He is also a Professor of Medical Physics at the University Medical Center of Groningen (The Netherlands). He received a Ph.D. and habilitation (PD) in medical physics from Geneva University for dissertations on Monte Carlo modelling and quantitative analysis in positron emission tomography. Dr. Zaidi is actively involved in developing imaging solutions for cutting-edge interdisciplinary biomedical research and clinical diagnosis in addition to lecturing undergraduate and postgraduate courses on medical physics and medical imaging. His research is supported by the Swiss National Foundation and centres on modelling nuclear medical imaging systems using the Monte Carlo method, dosimetry, image correction, reconstruction and quantification techniques in emission tomography as well as statistical image analysis in molecular brain imaging, and more recently on novel design of dedicated high-resolution PET and combined PET-MR scanners. He was guest editor for 7 special issues of peer-reviewed journals dedicated to Medical Image Segmentation, PET Instrumentation and Novel Quantitative Techniques, Computational Anthropomorphic Anatomical Models, Respiratory and Cardiac Gating in PET Imaging, Evolving medical imaging techniques and Trends in PET quantification (2 parts) and serves as Past Editor-in-Chief for the Open Medical Imaging Journal, Deputy Editor for the British Journal of Radiology, Associate editor for Medical Physics, the International Journal of Biomedical Imaging, the International Journal of Tomography & Simulation and the Journal of Engineering & Applied Sciences. He is also a member of the editorial board of Nuclear Medicine Communications, Computer Methods and Programs in Biomedicine, International Journal of Molecular Imaging, Biomedical Imaging and Intervention Journal, the American Journal of Cancer Science, the Open Medical Imaging Journal, the Open Neuroimaging Journal, International Journal of Biomedical Engineering and Consumer Health Informatics and Recent Patents on Medical Imaging and serves as scientific reviewer for leading journals in medical imaging. He is a senior member of the IEEE and liaison representative of the International Organization for Medical Physics (IOMP) to the World Health Organization (WHO) in addition to being affiliated to several International medical physics and nuclear medicine organisations. He is involved in the evaluation of research proposals for European and International granting organisations and participates in the organisation of International symposia and top conferences as member of scientific committees. His academic accomplishments in the area of quantitative PET imaging have been well recognized by his peers and by the medical imaging community at large since he is a recipient of many awards and distinctions among which the prestigious 2003 Young Investigator Medical Imaging Science Award given by the Nuclear Medical and Imaging Sciences Technical Committee of the IEEE, the 2004 Mark Tetalman Memorial Award given by the Society of Nuclear Medicine, the 2007 Young Scientist Prize in Biological Physics given by the International Union of Pure and Applied Physics (IUPAP), the prestigious 2010 Kuwait Prize of Applied Sciences (known as the Middle Eastern Nobel Prize) given by the Kuwait Foundation for the Advancement of Sciences (KFAS) for “outstanding accomplishments in Biomedical technology”, the 2013 John S. Laughlin Young Scientist Award given by the American Association of Physicists in Medicine (AAPM) and the 2013 Vikram Sarabhai Oration Award given by the Society of Nuclear Medicine (India). Dr. Zaidi has been an invited speaker of many keynote lectures at an International level, has authored over 380 publications, including ~170 peer-reviewed journal articles, conference proceedings and book chapters and is the editor of three textbooks on Therapeutic Applications of Monte Carlo Calculations in Nuclear Medicine, Quantitative Analysis in Nuclear Medicine Imaging and Multimodality Molecular Imaging of Small Animals.
Speakers: Vasileios Mezaris (Information Technologies Institute / CERTH), Benoit Huet (Eurecom)
Location: Dupin 2
Presentations: Part A, Part B, Part C
Description:
The tremendous and continuously accelerated growth in the amount of images and videos on the cloud, together with the widespread availability of a wide range of non-PC end-user connected devices (ranging from smartphones and tablets to internet-enabled TV sets), is changing the ways in which people consume visual content. In this environment, the traditional PC-era visual- or text-based search paradigms do not fully meet the expectations of the users, who find it increasingly difficult to navigate in a sea of disassociated video content and discover scattered content items of various origin that may be able to serve their specific information needs, e.g. more information on a story that was briefly presented earlier in the news, or further footage of an object of desire that they just saw in a video. Inspired by the ubiquitous text-based hyperlinks and the way that text hyperlinking has transformed how people navigate through textual and other information, its analogous for video content, i.e. video hyperlinking, is emerging as a promising approach to making video more easily accessible and consumable.
Video hyperlinking is the introduction of links that originate from meaningful fragments of video content (e.g. a video shot) and point to other relevant content (which may be visual content, e.g. another video or a segment of it, or any other form of content, e.g. an audio recording or a relevant Wikipedia article)—just like traditional hyperlinks originate from meaningful parts of a text (e.g. a key-phrase or a name) and point to other related resources. However, the manual insertion of such links in newly-created videos is a form of content curation that very few content creators would be willing to perform and maintain over time, because of being an undeniably tedious process. What is needed for making hypervideo feasible is the development of methods for the automatic or semi-automatic identification of related content and the generation of the corresponding links, transforming the present day’s disassociated videos in the cloud into a connected and easy to navigate hypervideo collection. This brings new challenges in automatically processing the visual content and understanding the information it conveys at different granularities, in processing associated audio and textual information, and in intelligently exploiting all these analysis results for creating meaningful video hyperlinks. It also raises important questions concerning the granularities that are most appropriate for decomposing and linking the video content.
This tutorial will introduce the vision of hypervideo, and then focus on two equally important directions: the video (and associated information) analysis that is needed for supporting video hyperlinking; and, the ways for making use of these imperfect analysis results so as to effectively discover and establish meaningful video links at suitable granularity levels.
Biographies:
Vasileios Mezaris received the Diploma and Ph.D. degree in electrical and computer engineering from the Aristotle University of Thessaloniki, Thessaloniki, Greece, in 2001 and 2005, respectively. He is currently a Senior Researcher (Researcher B) with the Information Technologies Institute/Centre for Research and Technology Hellas, Thessaloniki. He has co-authored 24 papers in refereed international journals, 9 book chapters, and more than 80 papers in international conferences. He holds two patents. His current research interests include image and video analysis, event detection in multimedia, machine learning for multimedia analysis, contentbased and semantic image and video retrieval, medical image analysis. Dr. Mezaris is currently an Associate Editor of the IEEE Transactions on Multimedia.
Benoit Huet is Associate Professor in the multimedia information processing group of Eurecom (France). He received his BSc degree in computer science and engineering from the École Supérieure de Technologie Électrique (Groupe ESIEE, France) in 1992. In 1993, he was awarded the MSc degree in Artificial Intelligence from the University of Westminster (UK) with distinction, where he then spent two years working as a research and teaching assistant. He received his DPhil degree in Computer Science from the University of York (UK) for his research on the topic of object recognition from large databases. He was awarded the HDR (Habilitation to Direct Research) from the University of Nice Sophia Antipolis, France, in October 2012 on the topic of Multimedia Content Understanding: Bringing Context to Content. He is associate editor for Multimedia Tools and Application (Springer) and Multimedia Systems (Springer) and has been guest editor for a number of special issues (EURASIP Journal on Image and Video Processing, IEEE Multimedia). He regularly serves on the technical program committee of the top conference of the field (ACM MM/ICMR, IEEE ICME). He is chairing the IEEE MMTC Interest Group on Visual Analysis, Interaction and Content Management (VAIG). He is vice-chair of the IAPR Technical Committee 14 Signal Analysis for Machine Intelligence. His research interests include computer vision, large-scale multimedia data mining and indexing (still and/or moving images), content-based retrieval, semantic labelling and annotation of multimedia content, multimodal fusion, and pattern recognition.
Speakers: Hamid Krim (North Carolina State University) and A. Ben Hamza (Concordia University)
Location: Dickens 1+2
Presentation: here
Description:
In recent years, there has been a tremendous interest in developing computational geometric and topological methods for solving challenging signal and image analysis problems arising in a wide range of areas, including medicine, remote sensing, astronomy, robotics, security and defense. This has been motivated, in large part, by the fact that virtually all objects contain geometric and topological information. However, our awareness of the importance of this information has been an evolutionary development. In this tutorial, we aim at bringing the recent developments in the fast-growing field of geometric and topological computing by presenting a balanced coverage of both theoretical and practical issues. This important research field enjoys not only a broad and solid foundation upon which its future can be securely built, but it also offers great opportunities for researchers and practitioners to employ and integrate theories from geometry and topology and draw on established theoretical and algorithmic frameworks. To uncover key geometric and topological information from signals and images, we present in this tutorial several methods that combine classical mathematical machinery from geometry and algebraic topology with more recent algorithmic tools. We demonstrate the effectiveness of the presented approaches with an assortment of substantiating examples and experimental results. In addition, various applications to imaging, computer graphics and sensor networks will be discussed and illustrated.
While the geometric and topological tools for data analysis are becoming crucially important, as linear methods have run their course in applications, engineers, who generally are not provided the training in such tools, are increasingly aware of their need, without the normally very long time investment required from mathematics. Engineers are also superb at learning by examples accompanied with the theory pitched at the proper level. It is our goal to concentrate on the physical meaning of many of the non-Euclidean geometry and topology, without losing the rigor required for deeper development and exploration. In particular, we first discuss the limitation of the Euclidian geometry in capturing complete information about the real world setting of many, if not all, practical applications of interest to engineers and scientists in information sciences. We subsequently discuss the Riemannian Geometry in the context of applications to better illustrate the many concepts most applied scientists view as too terse or abstract. We expect the attendants to leave with a good working knowledge of these numerous concepts and will make available much of the software used to illustrate the ideas.
Biographies:
Hamid Krim received his degrees in electrical engineering. As a member of technical staff at AT&T Bell Labs, he has worked in the area of telephony and digital communication systems/subsystems. In 1991 he became a NSF Post-doctoral scholar at Foreign Centers of Excellence (LSS Sup-elec/University of Orsay, Paris, France). He subsequently joined the Laboratory for Information and Decision Systems, MIT, Cambridge, MA, as a Research Scientist performing/supervising research in his area of interest, and later as a faculty in the ECE department at North Carolina State University in Raleigh, N.C. in 1998. He is an original contributor and now an affiliate of the Center for Imaging Science sponsored by the Army. He also is a recipient of the NSF Career Young Investigator award. He is on the editorial board of the IEEE Transactions on Signal Processing and regularly contributes to the society in a variety of ways. His research interests are in statistical estimation and detection and mathematical modeling with a keen emphasis on applications.
A. Ben Hamza received the Ph.D. degree in electrical engineering from North Carolina State University, Raleigh, NC. He is an Associate Professor at the Concordia Institute for Information Systems Engineering (CIISE), Concordia University, Montreal, QC, Canada. Prior to joining CIISE, he was a postdoctoral researcher at Duke University, Durham, NC, affiliated with both the Department of Electrical and Computer Engineering and the Fitzpatrick Center for Photonics and Communications Systems. His current research interests include signal/image processing, computer graphics, multimedia security, and statistical quality assurance. Dr. Ben Hamza is a licensed Professional Engineer registered in Ontario.
Speakers: Aggelos K. Katsaggelos (Northwestern University), Jeremy Watt (Northwestern University)
Location: Dickens 3+4
Description:
Due to their wide applicability, sparse and low-rank models have quickly become some of the most important tools for today’s researchers in image/video processing, computer vision, machine learning, statistics, optimization, and bioinformatics. Application areas in which sparse and low-rank modelling tools have been applied span a wide range of topics in these fields including: image inpainting and compressive sensing, object/face recognition, clustering and classification, Deep Learning feature selection, Collaborative Filtering, video survelience, and many more.
However while sparse and low-rank models themselves are typically fairly straightforward to grasp in applications, often times the optimization machinery required to make use of these models can be unfamiliar to students and researchers with a more traditional electrical, biomedical, statistics, or computer engineering/science background. Therefore a major distinctive feature of this tutorial course will be a practical focus on connecting fundamental concepts in optimization with their natural (and cutting edge) extensions for solving sparse and low-rank problems. No previous exposure to nonlinear programming is required for this tutorial. We will review the fundamental concepts as needed throughout the course.
In this tutorial, based on a book manuscript currently under development, we will spend the first third of the class introducing sparse and low-rank models in the context of various applications. We will then spend the remainder of the class accessibly explaining the cutting edge methods used to solve sparse and low-rank recovery problems. This coverage of optimization techniques will include: a discussion of greedy methods for sparse recovery, an overview of essential concepts from nonlinear programming, smooth reformulation techniques for sparse and low-rank problems, accelerated proximal gradient techniques, and the Alternating Direction Method of Multipliers framework.
Biographies:
Aggelos K. Katsaggelos received the Diploma degree in electrical and mechanical engineering from the Aristotelian University of Thessaloniki, Greece, in 1979, and the M.S. and Ph.D. degrees in Electrical Engineering from the Georgia Institute of Technology, in 1981 and 1985, respectively. In 1985, he joined the Department of Electrical Engineering and Computer Science at Northwestern University, where he is currently a Professor holder of the AT&T chair. He heads the Image and Video Processing Laboratory at Northwestern University. He has published extensively in the areas of multimedia signal processing and communications (over 200 journal papers, 500 conference papers and 40 book chapters) and he is the holder of 20 international patents. He is the co-author of Rate-Distortion Based Video Compression (Kluwer, 1997), Super-Resolution for Images and Video (Claypool, 2007) and Joint Source-Channel Video Transmission (Claypool, 2007). Among his many professional activities Prof. Katsaggelos was Editor-in-Chief of the IEEE Signal Processing Magazine (1997–2002), a BOG Member of the IEEE Signal Processing Society (1999–2001), and a member of the Publication Board of the IEEE Proceedings (2003-2007). He is a Fellow of the IEEE (1998) and SPIE (2009) and the recipient of the IEEE Third Millennium Medal (2000), the IEEE Signal Processing Society Meritorious Service Award (2001), the IEEE Signal Processing Society Technical Achievement Award (2010), an IEEE Signal Processing Society Best Paper Award (2001), an IEEE ICME Paper Award (2006), an IEEE ICIP Paper Award (2007), an ISPA Paper Award (2009), and a EUSIPCO Paper Award (2013). He was a Distinguished Lecturer of the IEEE Signal Processing Society (2007–2008).
Jeremy Watt is currently a PhD candidate in Computer Science and Electrical Engineering in the EECS Department at Northwestern University. He holds a BS degree in Religious Studies and an MA in Pure Mathematics, both from Indiana University. His research focuses on optimization techniques for sparse and low-rank modeling as well as applications of these models to large-scale image/video processing and machine learning problems.
Speakers: Oscar Au (HKUST)
Location: Dickens 5+6
Description:
In March 2013, H.265/HEVC was completed and achieved its FDIS status. It is surely the most significant event in digital video compression field in a decade. With the collaborative effort of a lot of experts, H.265/HEVC can provide approximately twice the compression performance of prior standard, i.e. maintain the same level of video quality while using only half of the bit rate. In particular, it addresses a special emphasis on the hardware friendly design and parallel-processing architectures. Now the Joint Collaborative Team on Video Coding (JCTVC) is working hard on developing the extensions of H.265/HEVC to enhance the design and address different application scenarios (e.g. enhanced chroma formats, scalable video coding (SVC), and 3D applications).
In this tutorial, we will first give an introduction of the history of image/video coding including JPEG, MPEG-1, H.261, MPEG-2, H.263, MPEG-4, and H.264/AVC, and the related coding principles. Then we will describe the development of H.265/HEVC and the main coding tools that are accepted. We also examine some coding tools not accepted, and hotly discussed topics, which aroused a lot of attention and study during the meetings. There are plenty of research opportunities in H.265/HEVC and beyond. Participants will gain an understanding of novel techniques in the next generation video coding standards, along with some perspectives for the future applications and research opportunities. We will briefly describe the development status of SVC extension and 3D extension of HEVC.
Biography:
Oscar Au received his B.A.Sc. from Univ. of Toronto in 1986, his M.A. and Ph.D. from Princeton Univ. in 1988 and 1991 respectively. After being a postdoctoral researcher in Princeton Univ. for one year, he joined the Hong Kong University of Science and Technology (HKUST) as an Assistant Professor in 1992. He is/has been a Professor of the Dept. of Electronic and Computer Engineering, Director of Multimedia Technology Research Center (MTrec), and Director of the Computer Engineering (CPEG) Program in HKUST. His main research contributions are on video and image coding and processing, watermarking and light weight encryption, speech and audio processing. Research topics include fast motion estimation for MPEG-1/2/4, H.261/3/4 and AVS, optimal and fast sub-optimal rate control, mode decision, transcoding, denoising, deinterlacing, post-processing, multi-view coding, view interpolation, depth estimation, 3DTV, scalable video coding, distributed video coding, subpixel rendering, JPEG/JPEG2000, HDR imaging, compressive sensing, halftone image data hiding, GPU-processing, software-hardware co-design, etc. He has published 50+ technical journal papers, 320+ conference papers, and 70+ contributions to international standards. His fast motion estimation algorithms were accepted into the ISO/IEC 14496-7 MPEG-4 international video coding standard and the China AVS-M standard. His light-weight encryption and error resilience algorithms are accepted into the China AVS standard. He was Chair of Screen Content Coding AdHoc Group in the JCTVC for the ITU-T H.265 HEVC video coding standard. He has 18 granted US patents and is applying for 80+ more on his signal processing techniques. He has performed forensic investigation and stood as an expert witness in the Hong Kong courts many times. Dr. Au is a Fellow of the Institute of Electrical and Electronic Engineering (IEEE) and is a Board 0f Governor member of the Asia Pacific Signal and Information Processing Association (APSIPA). He is/was Associate Editors of IEEE Trans. On Circuits and Systems for Video Technology (TCSVT), IEEE Trans. on Image Processing (TIP), and IEEE Trans. on Circuits and Systems, Part 1 (TCAS1). He is on the Editorial Boards of Journal of Visual Communication and Image Representation (JVCIR), Journal of Signal Processing Systems (JSPS), APSIPA Trans. On Signal and Information Processing (TSIP), Journal of Multimedia (JMM), and Journal of Franklin Institute (JFI). He is/was Chair of IEEE CAS Technical Committee on Multimedia Systems and Applications (MSATC), Chair of SP TC on Multimedia Signal Processing (MMSP), and Chair of APSIPA TC on Image, Video and Multimedia (IVM). He is a member of CAS TC on Video Signal Processing and Communications (VSPC), CAS TC on Digital Signal Processing (DSP), SP TC on Image, Video and Multidimensional Signal Processing (IVMSP), SP TC on Information Forensics and Security (IFS), and ComSoc TC on Multimedia Communications (MMTC). He served on the Steering Committee of IEEE Trans. On Multimedia (TMM), and IEEE Int. Conf. of Multimedia and Expo (ICME). He also served on the organizing committee of IEEE Int. Symposium on Circuits and Systems (ISCAS) in 1997, IEEE Int. Conf. On Acoustics, Speech and Signal Processing (ICASSP) in 2003, the ISO/IEC MPEG 71st Meeting in 2005, Int. Conf. on Image Processing (ICIP) in 2010, and other conferences. He was General Chair of Pacific-Rim Conference on Multimedia (PCM) in 2007, IEEE Int. Conf. on Multimedia and Expo (ICME) in 2010 and the International Packet Video Workshop (PV) in 2010. He won best paper awards in SiPS 2007, PCM 2007 and MMSP 2012. He is an IEEE Distinguished Lecturer (DLP) in 2009 and 2010, and has been keynote speaker for multiple times.
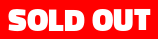
Speaker: Xiaogang Wang (The Chinese University of Hong Kong)
Location: Dickens 1+2
Presentation:

Description:
As a major breakthrough in artificial intelligence, deep learning has achieved very impressive success on solving grand challenges in many fields including computer vision and image and video processing. Deep models significantly advance the state-of-the-art in these challenges because of their ability to automatically learn hierarchical feature representations from data, to disentangle hidden factors, to jointly optimize key components in computer-vision, image-, and video-processing systems, and their large learning capacity. Deep learning has drawn broad interest from researchers in the fields of computer vision and image and video processing. We would like to share our research experience on how to see deep learning from the computer-vision and image-processing points of view.
In this tutorial, we will first give an overview of deep-learning research in the past years and introduce some classical deep models. Then we will focus on its applications to object detection, segmentation, and recognition. Through concrete examples in these applications, we will share our research experience on how to formulate a vision problem with deep learning and how to effectively train a deep neural network. Instead of treating a deep model as a black box, we investigate the connection between deep models and existing vision systems, such that a number of insights and experience accumulated from past vision research can be used to develop new deep models—including new layers and new architectures—and to design effective training strategies. Benefiting from the large learning capacity as well as the capability of disentangling multiple hidden factors in images hierarchically and nonlinearly, we can recast some classical object-detection, segmentation, and recognition problems as high-dimensional data-transform problems and solve them from a new perspective with deep models.
Biography:
Xiaogang Wang received the B.S. degree from University of Science and Technology of China in Electrical Engineering and Information Science in 2001 and the M.S. degree from Chinese University of Hong Kong in Information Engineering in 2004. He received the Ph.D. degree in Computer Science from the Massachusetts Institute of Technology. He is currently an assistant professor in the Department of Electronic Engineering at the Chinese University of Hong Kong. He was an Area Chair of IEEE International Conference on Computer Vision (ICCV) in 2011. He is an associate editor of the Image and Visual Computing Journal. He received the Outstanding Young Researcher in Automatic Human Behavior Analysis award in 2011 and the Hong Kong RGC Early Career Award in 2012. His research interests include computer vision and machine learning.
Speaker: Alessandro Foi (Tampere University of Technology)
Location: Dickens 3+4
Description:
The additive white Gaussian noise (AWGN) model is ubiquitous in signal processing. However, data acquired in real applications can seldom be described with good approximation by the AWGN model. Failure to model accurately the noise leads to misleading analysis, ineffective filtering, and distortion in the estimation. This tutorial provides an introduction to signal-dependent noise and to the models and methods for the practical processing of signals corrupted by such noise. Special emphasis is placed on effective techniques for noise suppression, and in particular on the recent developments in the optimal design of forward and inverse variance-stabilizing transformations. The distribution families covered as leading examples in the tutorial include Poisson, Rayleigh, Rice, multiplicative families, as well as doubly censored distributions. Consequently, the introduced models and techniques are applicable to several important signal processing scenarios, such as raw data from digital camera sensors, synthetic aperture radar imaging, ultrasound and seismic sensing, photon-limited imaging in astronomy and biomedical imaging, magnetic resonance imaging, etc. The tutorial is accompanied by numerous experimental examples where the presented methods are applied to competitive signal processing problems, often achieving the state of the art in image and multidimensional data restoration. Matlab software, which implements the presented techniques and experiments, is publicly available from the instructor’s website.
Biography:
Alessandro Foi received the M.Sc. degree from the Università degli Studi di Milano, Milan, Italy, and the Ph.D. degree from the Politecnico di Milano, Milan, in 2001 and 2005, respectively, both in mathematics, and the D.Sc.Tech. degree in signal processing from Tampere University of Technology, Tampere, Finland, in 2007. He is currently an Academy Research Fellow with the Academy of Finland, Department of Signal Processing, Tampere University of Technology. His recent work focuses on spatially adaptive (anisotropic, nonlocal) algorithms for the restoration and enhancement of digital images, noise modeling for imaging devices, and the optimal design of statistical transformations for the stabilization, normalization, and analysis of random data. His current research interests include mathematical and statistical methods for signal processing, functional and harmonic analysis, and computational modeling of the human visual systems. Dr. Foi is an associate editor for the IEEE Transactions on Image Processing.
Speaker: Ali C. Begen (Cisco)
Location: Dickens 5+6
Presentation:

Description:
This tutorial consists of two parts. In the first part, we provide a detailed overview of IPTV and its building blocks, explain the architectures and protocols used to carry video over IP in core, aggregation, access and home networks along with some observations and experiences from real deployments. In the second part, we survey well-established streaming solutions for over-the-top (OTT) video delivery, explaining how OTT video delivery contrasts to traditional broadcast and managed IPTV services. We then describe existing and emerging video service models reliant upon Internet video, including HTTP adaptive streaming with its all stages from content generation to distribution and consumption. Throughout the tutorial, we review recent research findings along with a discussion of future research directions.
Biography:
Ali C. Begen is with the Video and Content Platforms Research and Advanced Development Group at Cisco. His interests include networked entertainment, Internet multimedia, transport protocols and content delivery. Ali is currently working on architectures for next-generation video transport and distribution over IP networks, and he is an active contributor in the IETF and MPEG in these areas. Ali holds a Ph.D. degree in electrical and computer engineering from Georgia Tech. He received the Best Student-Paper Award at IEEE ICIP 2003, the Most-Cited Paper Award from Elsevier Signal Processing: Image Communication in 2008, and the Best-Paper Award at Packet Video Workshop 2012. Ali has been an editor for the Consumer Communications and Networking series in the IEEE Communications Magazine since 2011 and an associate editor for the IEEE Transactions on Multimedia since 2013. He is a senior member of the IEEE and a senior member of the ACM. Further information on Ali’s projects, publications, and presentations can be found at http://ali.begen.net.
Speakers: B. Ravi Kiran (ESIEE), Jean Serra (ESIEE), Jean Cousty (ESIEE), and Hughes Talbot (ESIEE)
Location: Dupin 1
Presentations: here
Description:
There has been increasing use of the hierarchical optimization paradigm in various problems, Hierarchical segmentation, and its evaluation using the Berkeley dataset, Total variation minimization, Hierarchical optical flow estimation methods, in the recent few years. Many multi-resolution signal decomposition and de-noising have inherent hierarchical structures. This tutorial provides an overview of the hierarchical optimization methods, defining the space of optimization to be a hierarchy of partitions. Many of these problems can be formulated and solved as an energy minimization over such hierarchical partitions structure. Moreover there is currently a growing interest for such questions, because they provide algorithms of reduced complexity. Hierarchies can describe some image, or some constraints and some parameters, which themselves turn out to be hierarchical. An example of the first case is given by the watershed trees, and second case by wavelets.
The tutorial will comprise of three parts. First the notion of hierarchy of partitions is defined and the various modes of representation are described. Then we will focus on various methods for generating hierarchies of partitions. In the last part several optimization methods are presented.
Biographies:
B. Ravi Kiran is a PhD Student at the A3SI group, ESIEE (École Supérieure d ‘Ingénieurs en Électronique et Électrotechnique) under University Paris-Est. He works in the problems of Optimization on hierarchies of segmentations, multi-variable optimization on hierarchies, and geospatial analysis. Before the PhD, he worked as a Project consultant at the Computer Vision and Artificial intelligence lab at the Indian Institute of Science (IISc) Bangalore, on the areas Road Segmentation for Driving assitance systems, Compressed domain motion segmentation and other video surveillance analytics. Before joining IISc, He worked at Texas instruments, Bangalore in the Video and Systems Engineering team.
Jean Serra co-founded the theory of Mathematical Morphology and in 1967, co-founded the Centre de Morphologie Mathématique, at School of Mines of Paris, which he led from 1979 to 2002. He is currently emeritus professor at the ESIEE Institute of Paris-Est University. He is the author or co-author of about two hundred scientific papers and of twelve books. His achievements also include several patents of devices for image processing. He founded the International Society for Mathematical Morphology in 1993, and was elected his first president. He also received various awards and titles, such as Doctor Honoris Causa of the Autonomous University of Barcelona (Spain) in 1993, and the first great prize of the French society for Pattern Recognition (AFFCET) in 1988. He was elected at the Royal Academy of Sciences of Uppsala, Sweden in 2006.
Jean Cousty received his Ingénieur’s degree from the École Supérieure d’Ingénieurs en Électrotechnique et Électronique (ESIEE Paris, France) in 2004 and the Ph.D. degree from the Université de Marne-la-Vallée (France) in 2007. During his PhD, he worked with Gilles Bertrand, Michel Couprie and Laurent Najman on the development of graph-based mathematical morphology and to its applications for cardiac image processing. For his PhD work, he received a special award from AFRIF association (French association for pattern recognition and interpretation). After a one-year post-doctoral period in the ASCLEPIOS research team at INRIA (Sophia-Antipolis, France), he is now teaching and doing research in the Computer Science and Telecom Department at ESIEE Paris. He is also a member of the Laboratoire d’Informatique Gaspard Monge at Université Paris-Est (joint research lab granted by CNRS, Université Paris-Est Marne-la-Vallée, ESIEE Paris and Ecole des Ponts Paritech). Since 2010, he is the co-head of the computer-science specialization at ESIEE Paris. His current research interests are discrete mathematics and their applications to image analysis, including theories, algorithms and applications of mathematical morphology with a particular emphasize on hierarchical segmentation methods. He co-authored more than 50 scientific publications and patents and he is the co-advisor of 3 PhD thesis.
Hugues Talbot graduated from Ecole Centrale de Paris in 1989, obtained the MSc from Université Pierre et Marie Curie in 1990 and the PhD from Ecole des Mines de Paris in 1993. He was a principal research scientist at CSIRO, Sydney, Australia, between 1994 and 2004. He is now an associate professor at Université Paris-Est / ESIEE in Paris, France, where he runs the bio-informatics/imaging track. He is the co-author or co-editor of 6 books. He has published over 140 articles in the area of image processing, image analysis and computer vision. He has supervised or co-supervised 11 PhDs so far. He is the recipient of several prizes including the DuPont innovation award in 2006 for his work in medical imaging. He has been a member of the steering committee for ISMM since 2002, and was the secretary of the Australian IAPR (APRS) between 2002 and 2004. His main interests include mathematical morphology, discrete geometry, combinatorial and continuous optimization.
Speaker: Tülay Adalı (University of Maryland Baltimore County)
Location: Dupin 2
Description:
Data-driven methods are based on a simple generative model and hence can minimize the underlying assumptions on the data. They have emerged as promising alternatives to the traditional model-based approaches in many applications where the unknown dynamics are hard to characterize. Independent component analysis (ICA), in particular, has been a popular data-driven approach and an active area of research. Starting from a simple linear mixing model and the assumption of statistical independence, one can recover a set of linearly-mixed components to within a scaling and permutation ambiguity. It has been successfully applied to numerous data analysis problems in areas as diverse as biomedicine, communications, finance, geophysics, and remote sensing.
Most of these problems require analysis of multiple sets of data that are either of the same type as in multi-subject data, or of different types and nature as in multi-modality data. For the analysis of multiple sets of data, various possibilities arise, such as performing ICA separately on each dataset. While simple and thus attractive, such an approach faces a number of practical challenges such as ordering the estimated components due to the permutation ambiguity, and more importantly, fails to take advantage of the statistical dependence across multiple datasets while performing the decomposition. Multi-set canonical correlation analysis (MCCA), by contrast, can take second-order-statistical information among multiple datasets into account and has found wide application in data-driven analysis. A recent generalization of ICA to multiple datasets—independent vector analysis (IVA)—provides a more attractive solution to the problem by fully exploiting dependence across multiple datasets. Because of the availability of this additional diversity—statistical property—IVA provides much better performance than performing ICA separately on each dataset, and can take all order-statistics into account, not only second-order-statistics like MCCA.
The goal of this tutorial is to introduce the basic theory and methods for blind source separation, both for single and multi-set analyses, and to introduce a unifying umbrella such that most of the methods introduced to date can be addressed as special cases. The talk will review not only ICA and IVA but also other related methods such as principal component analysis, partial least squares, and CCA/MCCA, discuss their relationships to each other, and will address modeling assumptions, conditions for identifiability of the models, large sample properties, and algorithms. The more general case that takes more than one type of diversity into account will be considered with emphasis on the use of both higher-order statistics and sample dependence. For all the approaches we consider, we will discuss different models for their application to medical image analysis and fusion, including many examples, and will emphasize key points that come up in these and similar applications, such as how and when it is important to optimize the performance of a given algorithm, what the best algorithm might be for a particular problem, and how to evaluate performance in a real application.
Biography:
Tülay Adalı received the Ph.D. degree in electrical engineering from North Carolina State University, Raleigh, in 1992 and joined the faculty at the University of Maryland Baltimore County (UMBC), Baltimore, the same year. She is currently a Professor in the Department of Computer Science and Electrical Engineering at UMBC. Prof. Adalı assisted in the organization of a number of international conferences and workshops including the IEEE International Conference on Acoustics, Speech, and Signal Processing (ICASSP), the IEEE International Workshop on Neural Networks for Signal Processing (NNSP), and the IEEE International Workshop on Machine Learning for Signal Processing (MLSP). She was the General Co-Chair, NNSP (2001–2003); Technical Chair, MLSP (2004–2008); Program Co-Chair, MLSP (2008 and 2009), 2009 International Conference on Independent Component Analysis and Source Separation; Publicity Chair, ICASSP (2000 and 2005); and Publications Co-Chair, ICASSP 2008. Prof. Adalı chaired the IEEE Signal Processing Society (SPS) MLSP Technical Committee (2003–2005, 2011–2013), served on the SPS Conference Board (1998–2006), and the Bio Imaging and Signal Processing Technical Committee (2004–2007). She was an Associate Editor for IEEE Transactions on Signal Processing (2003–2006), IEEE Transactions on Biomedical Engineering (2007–2013), IEEE Journal Of Selected Areas In Signal Processing (2010–2013), and Elsevier Signal Processing Journal (2007–2010). She is currently serving on the Editorial Boards of the Proceedings of the IEEE and Journal of Signal Processing Systems for Signal, Image, and Video Technology, and is a member of the IEEE SPS MLSP and Signal Processing Theory and Methods Technical Committees. Prof. Adalı is a Fellow of the IEEE and the AIMBE, recipient of a 2010 IEEE Signal Processing Society Best Paper Award, 2013 University System of Maryland Regents’ Award for Research, and an NSF CAREER Award. She is an IEEE Signal Processing Society Distinguished Lecturer for 2012 and 2013. Her research interests are in the areas of statistical signal processing, machine learning for signal processing, and biomedical data analysis.